Abstract
This article offers examples of how modeling can motivate health equity inquiry and research. This article also considers how equity fits into cost-effectiveness frameworks, how economic modeling can broaden the range of options for improving health equity, and how information other than results of cost- effectiveness analyses can inform health technology assessment.
Cost-Effectiveness Analyses
Cost-effectiveness analysis (CEA) is an important method for determining the relative efficiency of different ways of meeting an objective (eg, maximizing population health). Economic decision modeling creates CEAs for applied health technology assessment processes that make funding recommendations about health care innovations for populations (ie, not for a patient or a physician deciding on a treatment). Modeling allows analysts to go beyond the data, extrapolating to different populations using different outcomes over longer time horizons. For example, the Institute for Clinical and Economic Review (ICER) recently evaluated 2 new drugs for the treatment of hereditary transthyretin amyloidosis (hATTR), a rare disease with high unmet need.1 Using decision modeling, the research team considered the value of the new drugs in terms of both costs and quality-adjusted life-years over the lifetime of patients with hATTR.2
ICER’s independent voting panels consider not only cost-effectiveness but also other benefits and contextual considerations, such as social and environmental factors, when assessing the long-term value of new treatments. This process is an inconvenient reality for people hoping for a simple, one-dimensional way to assess value. Recent research by Harvard et al3 reviewed philosophical arguments on the influence of social values throughout scientific inquiry and applied them to better understand where value judgments occur in economic decision modeling. In our paper, we consider practical examples of opportunities for decision modeling to facilitate health equity considerations. Our first example considers how equity concerns fit into a standard cost-effectiveness framework. Next, we consider how economic modeling can broaden the scope of the options for improving health equity by utilizing nonhealth care-related resources. Lastly, we explore the impact on applied health technology assessment of considering other information in addition to CEA results.
Prioritizing Equity in Cost-Effectiveness
In addition to insufficient information to measure the trade-off between efficiency and equity,4 another challenge in choosing “reduction of inequity” as the objective in a CEA is that one must define equity (in order to know if it has been reduced).5,6 As Gravelle et al note,7 the concept of inequity is linked to need and “there is considerable debate about the meaning of ‘need’ and whether equity concerns should relate to health status, the amount of health care received, or access to health care.” After assuming that “need” has been neatly defined, the 2 types of equity challenges in allocating resources are horizontal inequity and vertical inequity.7 Figure 1 illustrates both.
Figure 1. Mismatch Between Care and Need in Vertical and Horizontal Inequity Problems
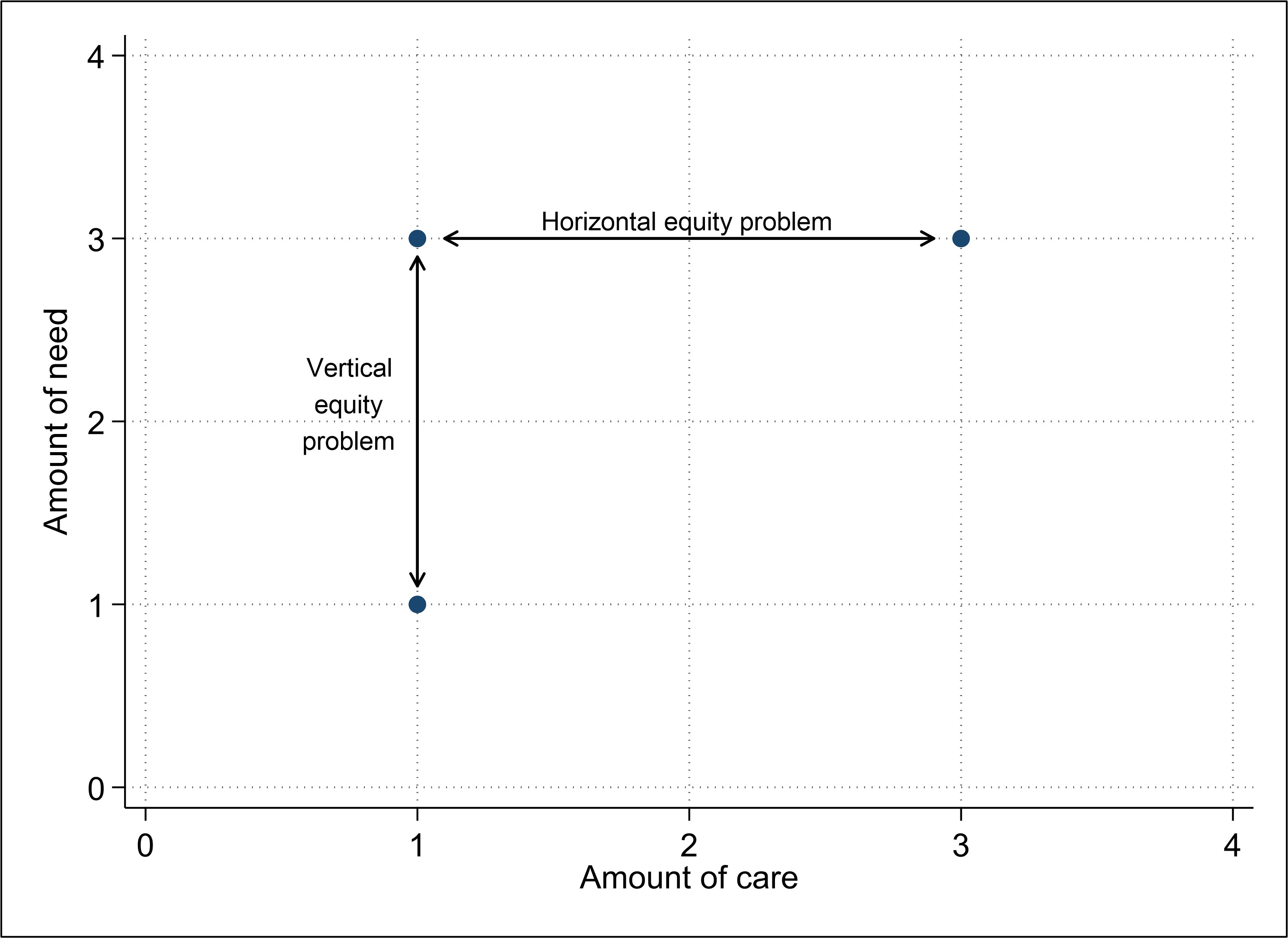
With horizontal inequity (shown horizontally on a hypothetical scale from 0 to 4, chosen for convenience), people with the same level of need get different amounts of health care (referred to as care subsequently). In contrast, with vertical inequity (shown vertically on a hypothetical scale from 0 to 4, chosen for convenience), people with different amounts of need get the same amount of care. It is not clear from Figure 1 in which direction the plotted dots should move to efficiently allocate limited resources. When amounts of care differ (different horizontal position), is inequity more efficiently reduced with more or less care? Likewise, when there are different amounts of need (different vertical position), is inequity more efficiently reduced with more or less care?
Figure 2 presents the optimal combinations of care (illustrated with a dashed line) as a function of need. Figure 2’s axes are reversed from Figure 1 so that a higher or lower amount of care is illustrated in an intuitive fashion.
Figure 2. Three Strategies to Address Vertical and Horizontal Inequity Problems
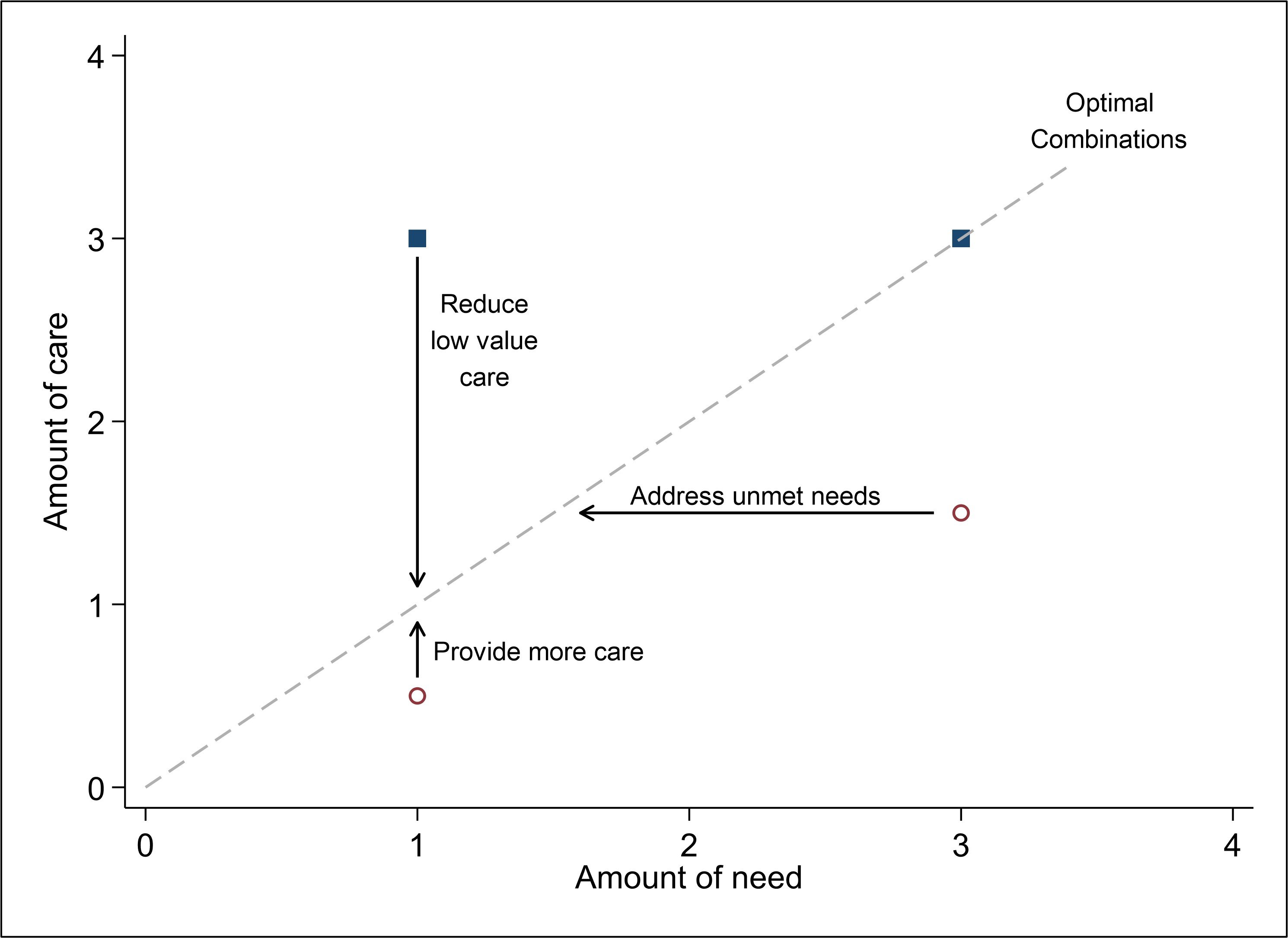
Modeling facilitates health equity considerations by identifying which options should be considered and which should be ruled out. For patients with low need who are receiving too little care (ie, the bottom left ¡ in Figure 2), raising the amount of care is optimal. However, the vertical equity problem illustrated by the n’s in Figure 2 can be efficiently addressed by actually reducing the amount of low-value care to the level indicated by the dashed line. This observation is a reminder that when optimizing care with respect to need, although it may be commonplace to think first of providing more care to those most in need, addressing the issues of people with less need may be both more feasible and more impactful. Interventions reducing low-value care for people who have low need might be less expensive (eg, less investment required) and more impactful (eg, more people affected). For low-need patients who are receiving more care than is optimal—such as more tests being ordered than needed—focusing on value instead of volume has the potential to free up scarce resources. Potentially, these resources could then be used to provide additional care for those in greater need. In theory, Figure 2 also illustrates that a more optimal care-need balance for “high-need” patients can be obtained by reducing their needs (instead of or in addition to by providing more care). The dashed optimality line is useful in decision making, as it suggests a way to spend limited resources efficiently to achieve maximum impact. In addition, decision modeling can help determine the dashed optimality line when clinical guidelines include cost-effectiveness information.8
Broadening the Option Range
While there are many ways of achieving greater health equity, nonhealth interventions, such as those that address social determinants of health, can be very cost-effective. Indeed, providing housing, food, and safety interventions can be more effective in reducing health inequity than providing more health care. Economic modeling can broaden the scope of the options considered since there is no restriction on which option to study; thus, by examining the value of any option (eg, a social intervention), health equity could be improved. For example, a recent modeling analysis9 of options to help homeless individuals considered nonclinical options like housing. When viewed within the framework illustrated in Figure 2, providing housing reduces need (ie, moves people to the left along the horizontal axis). A systematic review10 of Housing First programs, which provides housing with support services, concluded that while these programs cannot be expected to pay for themselves, “they represent a more efficient allocation of resources than traditional services.” This suggests that the most economically efficient way for a system to cope with homeless people overwhelming emergency rooms may not be to invest in more health care capacity but to reduce need through other social services. Of course, increasing social services will increase costs in a different sector.
CEAs of harm prevention strategies provide another example of how modeling supports the value of nontraditional options for helping vulnerable people. Although harm prevention strategies often involve options that some may find counterintuitive, modeling can show that they are cost-effective in terms of their efficiency in meeting a stated objective. For example, studies of supervised injection facilities have found that they are economically attractive,11 especially when the goal is to increase quality-adjusted life-years.12 However, stigmatization of programs such as these often prevent individuals from considering their cost-effectiveness.13
Applied Health Technology
When applied health technology assessment processes incorporate evidence to inform funding recommendations, different values, including health equity concerns, come into play. For example, Trenaman et al14 reviewed ICER’s health technology assessments and described how cost-effectiveness, other benefits or disadvantages, and contextual considerations affected voting members’ assessments of value. On average, new interventions with more attractive cost-effectiveness results received higher proportions of “high and intermediate” value votes. Among interventions with “other benefits” supporting a product’s value, having a novel mechanism of action (eg, the first generation of a therapy) received the most votes and reducing health disparities received the fewest, possibly because estimates of the health- and nonhealth-related opportunity costs were not available. The results thus highlight that factors beyond cost-effectiveness can lead to lower or higher assessments of value. ICER’s use of economic decision modeling indicates that “reducing health disparities” is an explicit consideration, given its categorization as one of the other benefits to be considered during each value vote. However, the fact that it received a small number of value votes in ICER’s health technology assessments suggests that new health care treatments may not be a common way to remedy health inequity.
Conclusion
Economic decision modeling can facilitate health equity considerations through its design and use.15,16 Framing a cost-effectiveness analysis involves focusing on the structure of the problems and on options, objectives, and costs. Using modeling to study the relative value of different options, including reduction of health inequity, can motivate the “business case” for different types of investments. Modeling allows one to consider different ways of addressing health inequity, which is an important advantage, given that health inequity wounds may be healed by improvements in areas in addition to health care.17 However, in countries where health care providers seek to maximize profit, one must be able to make the business case that one can do well by doing good. Modeling provides an important input into this process by careful consideration of different options, outcomes, and perspectives. Once the analysis identifies the potential interventions that target social determinants of health, negotiations can begin with a trusted broker to ensure it is worth everyone’s while to aid those who most need help.18
References
- Mickle K, Lasser KE, Hoch JS, Cipriano LE, Dreitlein WB, Pearson SD. The effectiveness and value of patisiran and inotersen for hereditary transthyretin amyloidosis. J Manag Care Spec Pharm. 2019;25(1):10-15.
-
Lasser KE, Hoch JS, Mickle K, et al. Inotersen and Patisiran for Hereditary Transthyretin Amyloidosis: Effectiveness and Value. Final Evidence Report. Institute for Clinical and Economic Review. October 4, 2018. Accessed December 17, 2020. https://collections.nlm.nih.gov/master/borndig/101745015/ICER_Amyloidosis_Final_Evidence_Report_101718.pdf
-
Harvard S, Werker GR, Silva DS. Social, ethical, and other value judgments in health economics modelling. Soc Sci Med. 2020;253:112975.
-
Sandiford P, Vivas Consuelo D, Rouse P, Bramley D. The trade-off between equity and efficiency in population health gain: making it real. Soc Sci Med. 2018;212:136-144.
-
Wagstaff A, van Doorslaer E. Equity in health care finance and delivery. In: Culyer AJ, Newhouse JP, eds. Handbook of Health Economics. Elsevier Science; 2000:1803-1862.
-
Williams A, Cookson R. Equity in health. In: Culyer AJ, Newhouse JP, eds. Handbook of Health Economics. Elsevier Science; 2000:1863-1910.
-
Gravelle H, Morris S, Sutton M. Economic studies of equity in the consumption of health care. In: Jones A, ed. The Elgar Companion to Health Economics. Edward Elgar Publishing; 2006:193-218.
-
Hummel EK, Ubel PA. Cost and clinical practice guidelines: can two wrongs make it right? Virtual Mentor. 2004;6(12):558-560.
-
Hoch JS, Trenaman L. Economic analysis of options for helping people experiencing homelessness in Sacramento. Center for Healthcare Policy and Research, University of California, Davis; 2020. Accessed April 29, 2021. https://health.ucdavis.edu/chpr/policy/publications/downloads/Economic_Analysis_Report_Summer2020_FIN-1.pdf
- Ly A, Latimer E. Housing first impact on costs and associated cost offsets: a review of the literature. Can J Psychiatry. 2015;60(11):475-487.
- Bayoumi AM, Zaric GS. The cost-effectiveness of Vancouver’s supervised injection facility. CMAJ. 2008;179(11):1143-1151.
- Enns EA, Zaric GS, Strike CJ, Jairam JA, Kolla G, Bayoumi AM. Potential cost-effectiveness of supervised injection facilities in Toronto and Ottawa, Canada. Addiction. 2016;111(3):475-489.
- Des Jarlais DC, Arasteh K, Hagan H. Evaluating Vancouver’s supervised injection facility: data and dollars, symbols and ethics. CMAJ. 2008;179(11):1105-1106.
-
Trenaman L, Pearson SD, Hoch JS. How are incremental cost-effectiveness, contextual considerations, and other benefits viewed in health technology assessment recommendations in the United States? Value Health. 2020;23(5):576-584.
- Culyer AJ, Bombard Y. An equity framework for health technology assessments. Med Decis Making. 2012;32(3):428-441.
-
Benkhalti M, Espinoza M, Cookson R, Welch V, Tugwell P, Dagenais P. Development of a checklist to guide equity considerations in health technology assessment. Int J Technol Assess Health Care. 2021;37:e17.
-
National Academies of Sciences, Engineering, and Medicine. Measurement and health equity. In: Metrics That Matter for Population Health Action: Workshop Summary. National Academies Press; 2016:chap 4.
- Nichols LM, Taylor LA. Social determinants as public goods: a new approach to financing key investments in healthy communities. Health Aff (Millwood). 2018;37(8):1223-1230.