Abstract
Precision medicine research initiatives aim to use participants’ electronic health records (EHRs) to obtain rich longitudinal data for large-scale precision medicine studies. Although EHRs vary widely in their inclusion and formatting of social and behavioral data, these data are essential to investigating genetic and social factors in health disparities. We explore possible biases in collecting, using, and interpreting EHR-based social and behavioral data in precision medicine research and their consequences for health equity.
Social and Behavioral Data in Precision Medicine
“Precision medicine,” “individualized medicine,” and “personalized medicine” are terms used to describe the approach to health care that considers a broad range of data types to determine the unique treatment and care needs of an individual. While genomic variation has been a major focus of precision medicine, a number of programs recognize that moving toward truly personalized health care requires an understanding of biological, environmental, social, and behavioral determinants of health in addition to genomic data.1-7 Social and behavioral data cover a large range of information but generally can be grouped into 4 categories: demographic, lifestyle and behavioral, psychosocial, and geographic.8 The body of scientific research shows that inequalities in social conditions are fundamental causes of population health differences.9-11 Social and behavioral data are important in demonstrating the role of social conditions in these health differences. For example, factors such as substance use, eating habits, activity levels, and risk-taking behaviors account for approximately 40% to 50% of the risk associated with preventable premature deaths in the US.12,13
Currently, a number of large-scale cohort initiatives are collecting social and behavioral data for use in research.2-7 Until recently, these data have come from participant surveys and other retrospective self-report methods.2 However, many precision medicine research programs utilize electronic health record (EHR) data, as EHRs contain rich longitudinal and detailed phenotype data collected through patients’ visits.14,15 For research programs to improve health outcomes and address health disparities, social and behavioral data must be accurately collected from patients and be retrievable from EHRs.16 Currently, extraction and use of these data present challenges due to inconsistencies across EHRs and inaccuracies in recorded data. Unless these challenges are addressed, EHR-derived social and behavioral data could limit the usefulness and applicability of precision medicine research.
Thoughtful inquiry and expansive discourse on the limitations of EHR-derived social and behavioral data are necessary if precision medicine research initiatives are to avoid inadvertent harm. What data are included or excluded from EHRs that can impact the rigor of precision medicine research? How does bias occur in collecting, using, and interpreting social and behavioral data? What are the possible consequences of interpreting and using data gathered through biased collection methodologies? Grappling with these questions can help promote better understandings of the data’s limitations and help inform strategies to reduce the data’s misapplication by researchers.
Social and Behavioral Data Collection in EHRs
Recognizing the importance of formally and systematically capturing social and behavioral measures, the National Academy of Medicine (NAM) (formerly the Institute of Medicine) recommended social environment measures’ inclusion in EHRs.8 Specifically, the NAM recommends intentional collection of structured social environment data, which in turn would encourage standardization of such data across patients, thereby reducing the probability of undesired bias. The NAM also recommends that a plan be developed by the National Institutes of Health to expand the use of EHRs in research by including social and behavioral data.8 Recognition by the NAM of the need to incorporate social data in clinical care, together with the importance of these data in precision medicine research, is likely to accelerate inclusion of these data in EHRs.
Across most EHR platforms, however, patients’ social and behavioral data are not consistently collected. These data are often unstructured and highly variable,5 and their inclusion is at health care professionals’ discretion.6 An example of data from a hypothetical patient’s EHR is provided in the figure.
Figure. Example of Social and Behavioral Data That Might Be Included in a Patient’s EHR
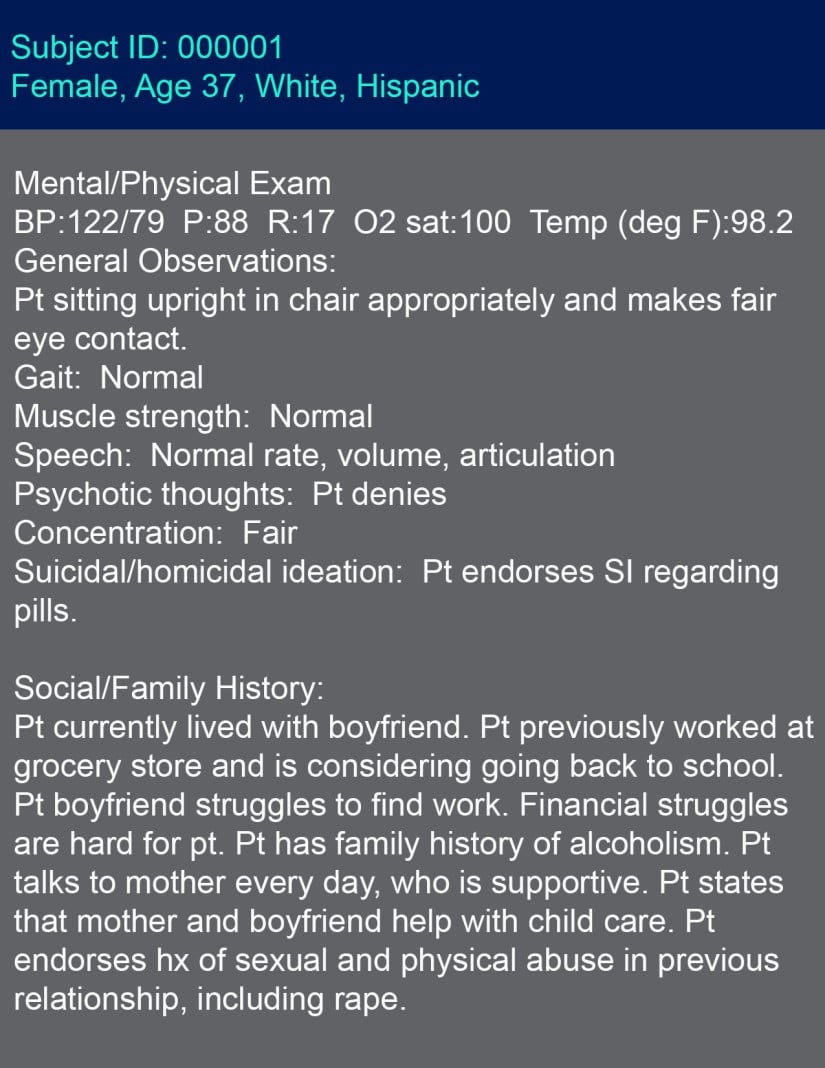
Variation in the content and completeness of social and behavioral data in EHRs is problematic for precision medicine research because the quality of the research is limited by the quality of the data. Despite challenges of uniform collection of social and behavioral data, methods are being developed to extract these data for use in large-scale precision medicine studies.17 As precision medicine research programs begin to utilize these data, it is important to consider the potential harms of their exclusion from EHRs or their misuse by researchers.
Limitations of Research with EHR Data
Limited patient participation. While EHR-derived social and behavioral data have potential to contribute to our understanding of multifactorial causes of health outcomes, which is one goal of precision medicine, these data require special consideration because they are commonly not self-reported by participants, who perceive such data as sensitive. Rather, clinicians normally record these data in patients’ EHRs. Potential study participants’ willingness to provide ongoing access to their EHR due to privacy concerns has been identified as a barrier to recruitment in precision medicine research programs.18
To encourage patient participation in precision medicine research programs that use EHR-derived social and behavioral data, it is important that researchers engage individuals as partners rather than only as prospective human subjects. Transparent communication and education about how participants’ information will be protected, deidentified, and used are imperative for maintaining trust so that individuals are more inclined to participate.15,19 It is important that potential participants understand how their data might be used, the limitations of privacy protections, and other potential risks.20
Due to the trust many physicians have established with their patients, they wield considerable influence on their patients’ decisions to participate in research. Trust between clinical professionals and their patients creates an environment wherein patients will be more likely to share their social and behavioral data for use in research. Consequently, physicians are likely to be key conduits for participant recruitment in precision medicine research programs.21 Therefore, it is up to these programs to develop relationships with physicians and keep them informed of, and involved with, precision medicine research programs.
Biases in collecting and analyzing EHR-derived social and behavioral data. Bias is present throughout the research process, from the recording of data to the interpretation of results. Decisions about which information to record in EHRs can lead to bias in the type of data available and affect the accuracy and completeness of what is recorded. For example, health care professionals, who vary in the content and completeness of data they include in EHRs,6 could be influenced by discussions of social and behavioral health indicators with patients, possibly unconsciously biasing available social and behavioral data. Because of data recording inconsistencies, important social and behavioral data could be missing from EHRs.17
Inclusion or exclusion of data from precision medicine studies can lead to confounding or misrepresenting research conclusions, which can be harmful in studies of diseases with health disparities.2 For example, in Non et al’s study of blood pressure, inclusion of education in the prediction model eliminated the association between genetic ancestry and blood pressure, since education was associated with both the predictor (genetic ancestry) and the outcome (blood pressure) variables.22 Exclusion of social and behavioral data from future precision medicine studies could generate misleading observations or spurious correlations between predictor and outcome variables.
Beyond biases in the recording of social and behavioral data, there can be biases in the use of these data by precision medicine researchers. When extracting social and behavioral data from unstructured free text rather than from structured fields of EHRs, methods such as text-mining algorithms are necessary. However, biases in the algorithm training data sets—for example, overrepresentation of a population—can lead to biases in the algorithms themselves, such that the algorithms only function for an overrepresented population.17
When social and behavioral data are missing, it can be challenging to determine how to approach large-scale analyses. Some methods for handling missing data, such as imputation, rely on creating new data from patterns in available data. But if the data used in imputation have biases, the imputed data will, too. Furthermore, most imputation methods developed for EHR data focus on clinical data. These methods are powerful but rely on assumptions of relationships between clinical variables such as hemoglobin A1c values and type 2 diabetes.23 Imputation methods can predict missing hemoglobin A1c values from available clinical data, such as diabetes medication use or fasting glucose measurements, because these values are clearly related.23 Given that imputation methods are prone to bias, imputed social and behavioral data might not be accurate because the relationships of social and behavioral variables to each other are less defined.
Another problematic approach is the use of EHR-derived social data without consideration of the social and historical biases inherent in the data’s collection.24,25 One example from outside of precision medicine is the use of policing data to build models of predictive policing. Data used in these models are based on existing patterns of police activity, which are already skewed due to overpolicing in minority neighborhoods. Therefore, when these models make recommendations for areas that require police monitoring, they utilize data that reinforce patterns of overpolicing.26 Within clinical settings, research has shown that EHR-derived data can be biased for several possible reasons, ranging from differences in physician delivery of care and recording of data to the methods of extracting the data from EHRs.27 When researchers make use of social and behavioral data in EHRs, it is important that they consider and are conscious of potential biases not only in the reporting of data but also in the extraction of data, in analyses, and in interpretation of results.28,29 Without addressing these considerations, models built on biased EHR-derived social and behavioral data may only reflect biases rather than useful information, as observed in the predictive policing example.
Within EHR research, frameworks for addressing bias for some types of clinical data have been developed; precision medicine researchers can utilize these methods when considering the biases of existing social and behavioral data in EHRs.30,31 Without carefully accounting for all sources of bias, precision medicine researchers have the potential to exacerbate the existing injustices that underrepresented populations experience.
Conclusion
The inclusion of EHR-derived social and behavioral data in precision medicine research is important to gain a holistic perspective of health. However, biases in the collection and analysis of EHR-derived social and behavioral data can have ethical implications. Researchers must use these data in a manner that will not exacerbate existing injustices in health care. Going forward, the inclusion of structured social and behavioral data in EHRs will aid in the process of reducing biases in documentation.
References
- Chambers DA, Feero WG, Khoury MJ. Convergence of implementation science, precision medicine, and the learning health care system: a new model for biomedical research. JAMA. 2016;315(18):1941-1942.
- Riley WT, Nilsen WJ, Manolio TA, Masys DR, Lauer M. News from the NIH: potential contributions of the behavioral and social sciences to the Precision Medicine Initiative. Transl Behav Med. 2015;5(3):249-246.
-
Gaziano JM, Concato J, Brophy M, et al. Million Veteran Program: A mega-biobank to study genetic influences on health and disease. J Clin Epidemiol. 2016;70:214-223.
-
Schaefer C; RPGEH GO Project Collaboration. C-A3-04: the Kaiser Permanente Research Program on Genes, Environment and Health: a resource for genetic epidemiology in adult health and aging. Clin Med Res. 2011;9(3-4):177-178.
-
Kaiser Permanente. Research program on genes, environment and health. https://divisionofresearch.kaiserpermanente.org/genetics/rpgeh/rpgehhome. Accessed July 13, 2018.
-
National Institutes of Health. All of Us research program website. https://allofus.nih.gov. Accessed July 13, 2018.
-
US Department of Veterans Affairs. Million Veteran Program. https://www.research.va.gov/mvp/. Accessed July 13, 2018.
-
Institute of Medicine. Capturing Social and Behavioral Domains and Measures in Electronic Health Records: Phase 2. Washington, DC: National Academies Press; 2015.
- Williams JS, Walker RJ, Egede LE. Achieving equity in an evolving healthcare system: opportunities and challenges. Am J Med Sci. 2016;351(1):33-43.
- Marmot M, Allen JJ. Social determinants of health equity. Am J Public Health. 2014;104(suppl 4):S517-S519.
-
Williams DR, Mohammed SA, Leavell J, Collins C. Race, socioeconomic status, and health: complexities, ongoing challenges, and research opportunities. Ann N Y Acad Sci. 2010;1186:69-101.
-
Nielsen L, Riddle M, King JW, et al. The NIH Science of Behavior Change Program: transforming the science through a focus on mechanisms of change. Behav Res Ther. 2018;101:3-11.
-
Crimmins EM, Preston SH, Cohen B, eds; National Research Council. International Differences in Mortality at Older Ages: Dimensions and Sources. Washington, DC: National Academies Press; 2010.
-
Wei WQ, Denny JC. Extracting research-quality phenotypes from electronic health records to support precision medicine. Genome Med. 2015;7(1):41. doi:10.1186/s13073-015-0166-y.
- Glicksberg BS, Johnson KW, Dudley JT. The next generation of precision medicine: observational studies, electronic health records, biobanks and continuous monitoring. Hum Mol Genet. 2018;27(R1):R56-r62.
-
Blizinsky KD, Bonham VL. Leveraging the learning health care model to improve equity in the age of genomic medicine. Learn Health Syst. 2018;2(1). doi:10.1002/lrh2.10046.
-
Hollister BM, Restrepo NA, Farber-Eger E, Crawford DC, Aldrich MC, Non A. Development and performance of text-mining algorithms to extract socioeconomic status from de-identified electronic health records. Pac Symp Biocomput. 2017;22:230-241.
-
Pittman D. Precision Medicine Initiative hits a snag. Politico. October 10, 2017. https://www.politico.com/tipsheets/morning-ehealth/2017/10/10/precision-medicine-initiative-hits-a-snag-222722. Accessed July 28, 2018.
- Sankar PL, Parker LS. The Precision Medicine Initiative’s All of Us research program: an agenda for research on its ethical, legal, and social issues. Genet Med. 2017;19(7):787-790.
- Adams SA, Petersen C. Precision medicine: opportunities, possibilities, and challenges for patients and providers. J Am Med Inform Assoc. 2016;23(4):787-790.
- Persaud A, Bonham VL. The role of the health care provider in building trust between patients and precision medicine research programs. Am J Bioeth. 2018;18(4):26-28.
- Non AL, Gravlee CC, Mulligan CJ. Education, genetic ancestry, and blood pressure in African Americans and Whites. Am J Public Health. 2012;102(8):1559-1565.
-
Wells BJ, Chagin KM, Nowacki AS, Kattan MW. Strategies for handling missing data in electronic health record derived data. EGEMS (Wash DC). 2013;1(3):1035. doi:10.13063/2327-9214.1035.
- Char DS, Shah NH, Magnus D. Implementing machine learning in health care—addressing ethical challenges. N Engl J Med. 2018;378(11):981-983.
-
Veale M, Binns R. Fairer machine learning in the real world: mitigating discrimination without collecting sensitive data. Big Data Soc. 2017;4(2). doi:10.1177/2053951717743530
-
Ensign D, Friedler SA, Neville S, Scheidegger C, Venkatasubramanian S. Runaway feedback loops in predictive policing. Proc Mach Learn Res. 2018;81:1-12.
-
Verheij RA, Curcin V, Delaney BC, McGilchrist MM. Possible sources of bias in primary care electronic health record data use and reuse. J Med Internet Res. 2018;20(5):e185. doi:10.2196/jmir.9134.
-
Sue S. Science, ethnicity, and bias: where have we gone wrong? Am Psychol. 1999;54(12):1070-1077.
- Moss-Racusin CA, Dovidio JF, Brescoll VL, Graham MJ, Handelsman J. Science faculty’s subtle gender biases favor male students. Proc Natl Acad Sci U S A. 2012;109(41):16474-16479.
- Bower JK, Patel S, Rudy JE, Felix AS. Addressing bias in electronic health record-based surveillance of cardiovascular disease risk: finding the signal through the noise. Curr Epidemiol Rep. 2017;4(4):346-352.
-
Haneuse S, Daniels M. A general framework for considering selection bias in EHR-based studies: what data are observed and why? EGEMS (Wash DC). 2016;4(1):1203. doi:10.13063/2327-9214.1203.