Abstract
Coded health care data from patients’ health records are used in epidemiological research, especially on incidence or prevalence of disease; for drug safety monitoring or long-term cohort tracking; and to inform policy making. This article briefly summarizes the evolution of internationally recognized coding ontologies and nomenclature and describes applications of coded electronic health record (EHR) data in day-to-day health care operations, research, auditing, and policy development. This article also illuminates how errors can occur when EHR information is coded, considers errors’ consequences, and suggests strategies for mitigating errors and improving overall use of coded EHR data.
A History of Health Care Data Coding
The classification or “coding” of diseases dates back to 17th-century England.1 At that time, codes were collected as part of the London Bills of Mortality to enable frequent causes of death to be recorded. While “Found dead in the Fields at St. Mary Islington”1 no longer has a code, a desire to capture such granularity in our health care systems remains today.
What would become known as the International Classification of Diseases (ICD) coding system was adopted by the International Statistical Institute in 1893, and diagnostic terms were introduced in the sixth revision of the ICD in 1948.2,3 Health care communities had recognized the ICD system officially before publication of the first volume of the ninth revision in 1977, at which point the ICD was expanded to include additional detail at the subcategory level. With each edition of the ICD, the number of codes increases, which facilitates billing and administration and the use of these data for audit and research purposes.
This article briefly summarizes the evolution of internationally recognized coding ontologies and nomenclature and describes applications of coded electronic health record (EHR) data in day-to-day health care operations, research, auditing, and policy development. This article also illuminates how errors can occur when EHR information is coded, considers errors’ consequences, and suggests strategies for mitigating errors and improving overall use of coded EHR data.
Types and Complexity of Codes
In addition to the ICD, other coding systems have evolved, the most commonly used of which is the SNOMED CT system, a consistent vocabulary for recording clinical information that is considered to be “the most comprehensive, multilingual clinical healthcare terminology” in existence.4 SNOMED CT was released in its current format in 2002 as a combination of reference terminology and clinical terms.5 The currently used coding systems in health care are summarized in the Table. It should be noted that individual ICD or SNOMED CT codes are added and retired over time, with the result that multiple codes exist to code for the same condition.6
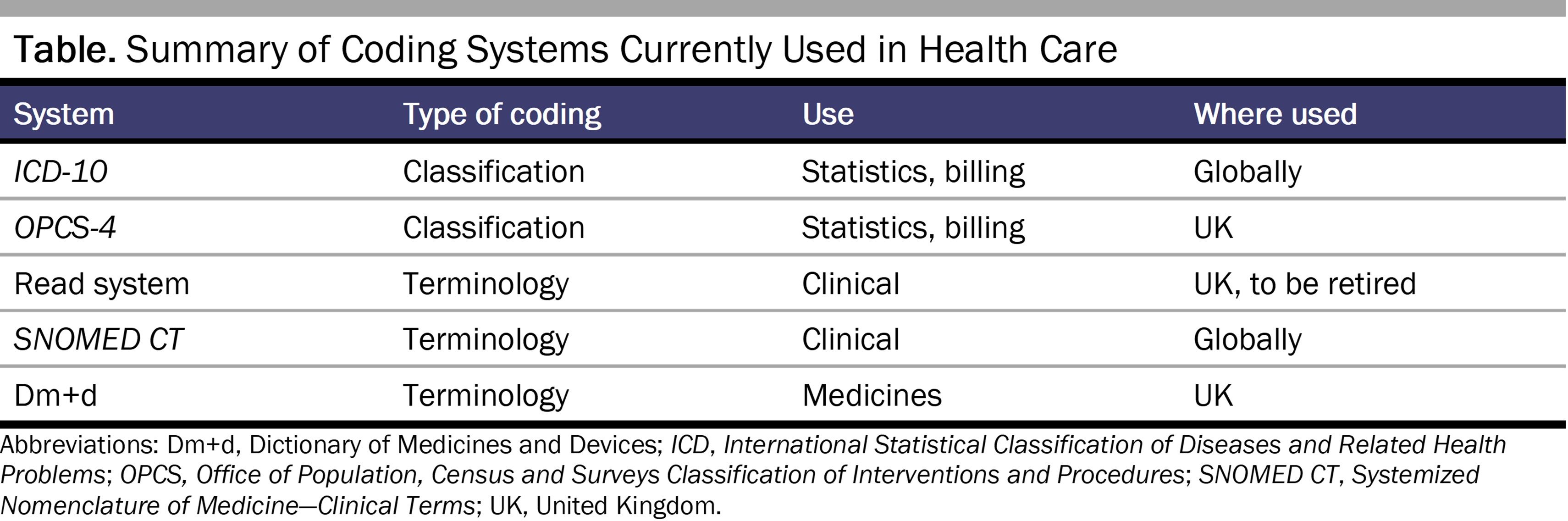
The complexity of coding is likely to increase, given that health care is increasingly reliant on technology and digital medical records. More data sources are becoming available (eg, patient-facing apps and wearable devices), which are linkable to other health care data sources that are accessible, both to patients and for research and policy making. This interconnectivity and accessibility make understanding of the use and accuracy of health care data all the more important. In addition, tools for using the data are becoming more complex, with artificial intelligence (AI) and machine learning algorithms that automate coding being used more frequently.7 Regardless of the methodology used, however, the accuracy of the coding underpinning EHR data is paramount to the data’s usefulness. There is a certain degree of false hope that AI will solve problems that current data strategies cannot (such as identifying individuals at high risk of disease), but the bottom line is that if the coding is not right to begin with, no amount of AI will make data analysis any better.
Beyond the importance of using data for day-to-day health care decisions for an individual, data are used for other reasons, ranging from monitoring quality of care and benchmarking services to measuring public health trends and disease epidemiology. Published papers using these data for research cover a wide variety of topics.8
Training Clinicians About Coding
In the United Kingdom (UK), medical coders undertake hospital coding, translating what is written in the medical records into ICD-10 codes, which are ultimately entered into hospital episode statistics (HES) and Office for National Statistics mortality data. HES are used by national bodies and regulators, including the Department of Health and Social Care and NHS England, for the purpose of health care analytics. The data are also available for research in deidentified format with appropriate permissions. There are strict rules concerning hospital coding and data entry, and, in the UK, medical coders are trained, as they are in other countries.9,10 Coders follow algorithms, which include instructions, such as coding a disease in place of symptoms in most cases; if a diagnosis is only possible, it cannot be coded, whereas if it is probable (not an impression or suspected), it can be coded. While there is clear guidance concerning what can be coded and how, there is too often little or no coordination between medical coders and medical staff, with coders having to interpret and decipher what has been written and medical staff not being aware of the nuances of coding rules.11,12 This compartmentalization can lead to inaccuracies in the data. One example is discrepancies in national respiratory audit data entry by clinicians and therefore spurious case ascertainment results. These discrepancies arise because data that do not meet inclusion criteria for the audit based on coding rules might be entered into the audit anyway by health care professionals.13 Ultimately, clinical staff are vital in ensuring accurate data acquisition and, ultimately, data quality.
In primary care in the UK, data entry is usually undertaken by health care professionals at the point of inputting the data during a consultation. Codes are often assigned via dropdown menus or attached to keywords in the background of the system. Even in this setting, however, as well as globally,14,15 health care professionals have minimal training as to the importance of choices of codes used or how they inform policy and contribute to audit and research. There is no formal requirement to teach UK doctors about coding classifications and terminologies, and a recent survey of UK medical schools found huge variation in the importance given to the area.16
Consequences of Coding Errors
At an individual level, inaccuracy in a person’s medical record can have significant consequences, and, in the UK, data once entered generally cannot be removed, although codes do exist to indicate a disease has resolved. For example, a patient’s record could contain a code for a disease they do not have, or there could be ambiguous granularity in diagnostic criteria that makes it difficult for new physicians seeing the patient to make decisions. Moreover, important aspects of care, such as identifying unpaid carers, is often not coded, thereby limiting offers of carer support. Errors can also be problematic at a system and population level.17
Knowledge and understanding of systems are essential for accurate use of health care data beyond clinical practice. Data may be missing from the EHR for a variety of reasons (eg, something is unknown or an individual declined to answer), which can introduce bias. Less obviously, health care professionals might be reluctant to code information related to wider determinants of health due to stigma or stereotyping and worries about how it might affect patients’ insurance coverage and job prospects. For example, health care professionals might be reluctant to code for a diagnosis, such as HIV, that the patient does not want to disclose if there is concern that insurers or employers could somehow find out about the diagnosis. Moreover, the variety of disease code sets used for clinical or billing purposes can result in different estimates of prevalence. Use of less accurate estimates for resource allocation planning can have a knock-on effect in terms of financial distributions that can ultimately be detrimental to patient care.18,19 Likewise, use of different disease code sets in research has resulted in mixed findings, such that associations between exposure and outcome variables are found to be present or not,20 and in the inability to make comparisons due to heterogeneity between coding systems. Inconsistency in results and, ultimately, variability of conclusions can undermine the value of these data for research.
Coding errors not only contribute to biased outcomes but have ethical implications if used by insurance or pharmaceutical companies for personal gain.21 Companies’ primary purpose, however, in using data from EHRs, pharmacy records, and billing and reimbursement documentation, is “to monitor medicine consumption and pharmaceutical spending, and to assess safety and providers’ compliance with guidelines.”22 Accurate and objective information is essential to guide policy making and spending and to avoid exacerbating health inequalities, lengthening waiting lists, and inappropriately prioritizing services. The earlier that data—and more complete data—can be made available, the more robust will be estimates and forecasts. However, politicization of epidemiological data can lead to misalignment of incentives and evaluations.23
Improving EHR Data Use
Ultimately, there needs to be trust in those using the data. Closer working relationships between health care professionals and medical coders and clinical ownership of codes and data are essential for mitigating errors and improving use of EHR data. Beyond individual efforts, there needs to be regulation and accreditation of health care data professionals and clearly defined roles for health care professionals in supplying context when inputting data. In research studies, reporting of codelists and of algorithms and methodology needs to be transparent so that analyses are reproducible. Audit programs are helpful for improving coding standards and could be undertaken as part of national audit programs for quality improvement. As with any research, integrity is key, and auditors need to be as transparent as possible. As a society, we also need to guard against people exploiting any uncertainty that arises from miscoding (or poor data quality) to advance their own agendas, which leads to a politicization (and mistrust) of health data.
In the same way that researchers would never undertake a clinical trial without clear definitions of endpoints, we should encourage consensus on and standardization of important disease endpoints for observational work using EHR data. Work has been undertaken to harmonize various coding ontologies by mapping to a common data model (eg, Observational Medical Outcomes Partnership), thereby allowing federated data analytics. While these efforts at standardization can speed up research and make cross-country or system comparisons easier to undertake, there is still potential for biased outcomes as the risk of cumulative errors and the complexity of the systems grows.
We must also accept that, in the UK, it will never be appropriate to remove information that has been entered in the EHR. In the same way that if we write something in error in a medical record, we cross it out and date and sign it, there are resolved codes that can be used in the EHR, but it would be inappropriate to ever delete something that has been included.
Conclusion
In the UK, we have moved from paper records to secure data environments in less than 15 years, which is relatively high speed, considering the complexity of health care. Most patients and the public are keen for their data to be used in health management so that it can be based on robust estimates of risk calculated from accurate, standardized data, although they may have questions about how the data will be used in research and by whom.24 Given that the data are imperfect, it is important for health care professionals to communicate any limitations, biases, and caveats that can originate from miscoding and that are relevant to day-to-day decision-making. From a public perspective, it is important that policy makers be provided with the highest-quality information to develop policy that prioritizes the right services for people who need them and reduces growing health inequalities.
Few doubt that clinical coding systems have led to improvements in health care research and provided benefits to patients and the public. They have allowed data to be linked at a personal level, enabled more detailed studies and standardized analytics, allowed for real-time analytics, and will provide training data for next-generation AI. Yet further improvements are needed. Standardization is becoming even more important, as once disparate data sources are being linked for federated analyses as part of national and international collaborations. Study findings can be influenced by lack of standardized coding and definitions, as well as by inclusion and exclusion criteria, missing data, and the like, and the effects of these factors are likely to be exacerbated if people train AI and use other new technologies without thorough testing, validation, and understanding of the algorithms. Accordingly, regulation, accreditation, and accountability will be important to maintain the integrity of health data and research.
References
- Boyce N. Bills of Mortality: tracking disease in early modern London. Lancet. 2020;395(10231):1186-1187.
-
Classifications and Terminologies Team. History of the development of the ICD. World Health Organization; 2021. Accessed August 20, 2024. https://cdn.who.int/media/docs/default-source/classification/icd/historyoficd.pdf
- Hirsch JA, Nicola G, McGinty G, et al. ICD-10: history and context. AJNR Am J Neuroradiol. 2016;37(4):596-599.
-
What is SNOMED CT? SNOMED International. Accessed October 3, 2024. https://www.snomed.org/what-is-snomed-ct
-
Overview of SNOMED CT. National Library of Medicine. Reviewed October 14, 2016. Accessed July 3, 2024. https://www.nlm.nih.gov/healthit/snomedct/snomed_overview.html
-
MacRae C, Whittaker H, Mukherjee M, et al. Deriving a standardised recommended respiratory disease codelist repository for future research. Pragmat Obs Res. 2022;13:1-8.
-
Dong H, Falis M, Whiteley W, et al. Automated clinical coding: what, why, and where we are? NPJ Digit Med. 2022;5(1):159.
- Cowie MR, Blomster JI, Curtis LH, et al. Electronic health records to facilitate clinical research. Clin Res Cardiol. 2017;106(1):1-9.
-
NCCQ. Institute of Health Records and Information Management. Accessed July 3, 2024. https://www.ihrim.co.uk/education-and-cpd/overseas-students/registration-forms/nccq
-
Building healthcare software—clinical coding, classifications and terminology. NHS England. Updated August 24, 2023. Accessed July 3, 2024. https://digital.nhs.uk/developer/guides-and-documentation/building-healthcare-software/clinical-coding-classifications-and-terminology
-
Terminology and Classifications Delivery Service. National Clinical Coding Standards ICD-10. 5th ed. NHS England; 2023. Accessed October 3, 2024. https://classbrowser.nhs.uk/ref_books/ICD-10_2023_5th_Ed_NCCS.pdf
-
ICD-10-CM Official Guidelines for Coding and Reporting. Centers for Medicare and Medicaid Services; 2021. Accessed July 3, 2024. https://www.cms.gov/files/document/2021-coding-guidelines-updated-12162020.pdf
-
Singh S, Legg M, Garnavos N, et al. National Asthma and Chronic Obstructive Pulmonary Disease Audit Programme (NACAP): pulmonary rehabilitation clinical audit 2019: clinical audit interim report. Royal College of Physicians; 2020. Accessed July 3, 2024. https://www.rcp.ac.uk/media/1tzfeeqi/nacap_prplusclinical_audit_report_julyplus2020_0.pdf
-
Alyahya MS, Khader YS. Health care professionals’ knowledge and awareness of the ICD-10 coding system for assigning the cause of perinatal deaths in Jordanian hospitals. J Multidiscip Healthc. 2019;12:149-157.
-
Asadi F, Afkhami S, Asadi F. Promotion of training course on ICD-10 poisoning coding: necessity to adopt preventive strategies. BMC Med Educ. 2023;23(1):903.
-
Health Data Research UK; Medical Schools Council; NHS England; NHS Education Scotland. Survey of data science in UK medical school curricula. Health Data Research UK; Medical Schools Council; 2023. Accessed August 27, 2024. https://www.hdruk.ac.uk/wp-content/uploads/2023/11/Survey-of-Data-Science-in-UK-Medical-School-Curricula-Report-August-2023.pdf
- Sauer CM, Chen LC, Hyland SL, Girbes A, Elbers P, Celi LA. Leveraging electronic health records for data science: common pitfalls and how to avoid them. Lancet Digit Health. 2022;4(12):e893-e898.
- Stone PW, Osen M, Ellis A, Coaker R, Quint JK. Prevalence of chronic obstructive pulmonary disease in England from 2000 to 2019. Int J Chron Obstruct Pulmon Dis. 2023;18(18):1565-1574.
-
Morgan A, Gupta RS, George PM, Quint JK. Validation of the recording of idiopathic pulmonary fibrosis in routinely collected electronic healthcare records in England. BMC Pulm Med. 2023;23(1):256.
-
Whittaker H, Rothnie KJ, Quint JK. Exploring the impact of varying definitions of exacerbations of chronic obstructive pulmonary disease in routinely collected electronic medical records. PLoS One. 2023;18(11):e0292876.
-
Neubert A, Brito Fernandes Ó, Lucevic A, et al. Understanding the use of patient-reported data by health care insurers: a scoping review. PLoS One. 2020;15(12):e0244546.
-
Using routinely collected data to inform pharmaceutical policies. OECD Web Archive. April 19, 2023. Accessed July 3, 2024. https://web-archive.oecd.org/2023-04-20/503807-routinely-collected-data-to-inform-pharmaceutical-policies.htm
- Wells CR, Galvani AP. Tackling the politicisation of COVID-19 data reporting through open access data sharing. Lancet Infect Dis. 2022;22(12):1660-1661.
-
Health data research explained. Health Data Research UK. Accessed July 3, 2024. https://www.hdruk.ac.uk/about-us/what-we-do/health-data-research-explained/